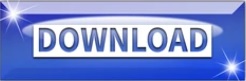

Whereas for optimization, the AI model already knows which precursors need to be added, and in which order. The difference being that, for discovery, the system is trying to determine which precursors need to be added, as well as the best order in which to add them, in order to find a chemistry with the best performance. This is true whether the system is focused on discovering a new chemical or optimizing the manufacturing process for a known chemical. "Basically, the system is capable of immediately learning from and adapting to unexpected results." From this, AlphaFlow can account for actions with delayed effects and also pivot its decision making based on the most recent experimental outcomes in real time," says Amanda Volk, first author of the paper and a Ph.D.
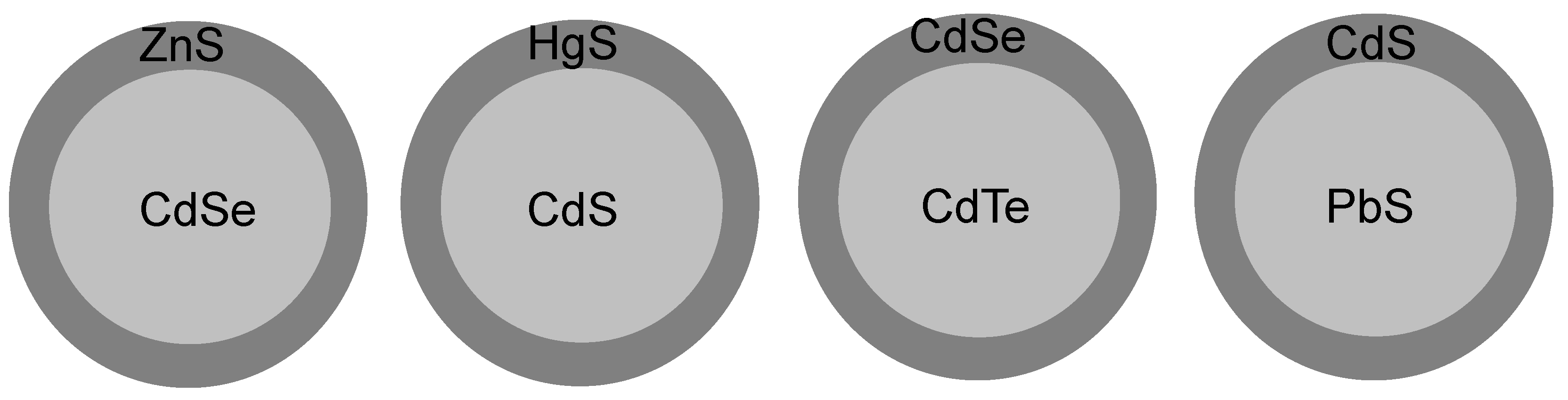
"We use this moving window of prior action steps and predicted outcomes of future action sequences to inform AlphaFlow's decision making. It's extremely efficient."ĪlphaFlow's AI model makes decisions on what experiment to conduct next based on two things: the data it has developed from experiments it already ran and what it predicts the results of the next several experiments will be. "It effectively miniaturizes the experiments and performs the same laboratory operations that would require an entire wet chemistry lab in a suitcase-sized end-to-end experimental platform. We've shown that AlphaFlow can conduct more experiments than 100 human chemists in the same period of time, while using less than 0.01% of the relevant chemicals." "Our system, called AlphaFlow, makes use of an artificial intelligence technique called reinforcement learning that-when coupled with automated microfluidic devices-expedites the material discovery process. "If a complex chemistry includes dozens of parameters, it might take decades to develop a new target material or a more efficient way to produce a desired chemical." "Progress in materials and molecular discovery is slow, because conventional techniques for discovering new chemistries rely on varying one parameter at a time using siloed operations in chemistry and materials science labs," says Milad Abolhasani, corresponding author of a paper on the work and a professor of chemical and biomolecular engineering at North Carolina State University. In a proof-of-concept demonstration, the system found a more efficient way to produce high-quality semiconductor nanocrystals that are used in optical and photonic devices. Credit: Nature Communications, DOI: 10.1038/s41469-yĪ team of chemical engineering researchers has developed a self-driven lab that is capable of identifying and optimizing new complex multistep reaction routes for the synthesis of advanced functional materials and molecules. Time estimates include preparation and sampling time and are based on 30 min to start each experiment and 5 min per addition of a reagent. Volume estimates are based on 10 ml starting material and an additional 5 ml of reagent per step. Reaction option estimates are based on four possible reagents, five possible volumes, and five possible reaction times. Illustration of the exponentially increasing complexity and required resources for a batch multi-step synthesis consisting of four possible step choices, up to 32 sequential steps. Curse of dimensionality in multi-step chemistry.
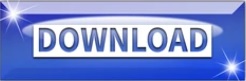